The meteorological field has made remarkable strides since its inception millennia ago when humans relied on 'cosmic signals' like lunar cycles, cloud patterns, and sky colors. From modern mathematical approaches to meteorological phenomena, the discipline has evolved significantly. Yet, regardless of the pinnacle of predictive technology, human intervention remains crucial for the most accurate conclusions.
Overall, commonplace weather events such as rain, tropical depressions, etc., can now be accurately forecasted using data aggregation techniques and calculations. For instance, rain prediction has been highly reliable since 1990, and the accuracy of predicting and monitoring tropical depressions has improved by over 50% in the last 30 years.
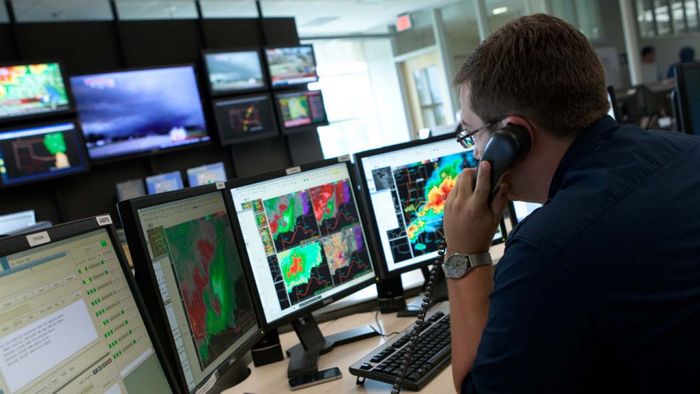
However, there's still an unpredictable phenomenon: thunderstorms, or storms characterized by thunder and lightning. Events like Typhoon Noru form abruptly, defying early detection and catching regions like the Philippines unprepared.
Utilizing AI and machine learning in hurricane predictionCurrently, the primary method for hydro-meteorological prediction relies on weather data models to make forecasts. These models operate by observing current weather conditions through weather stations, satellites, etc., to gather and 'decode' the atmospheric motion formulae for weather conclusions.
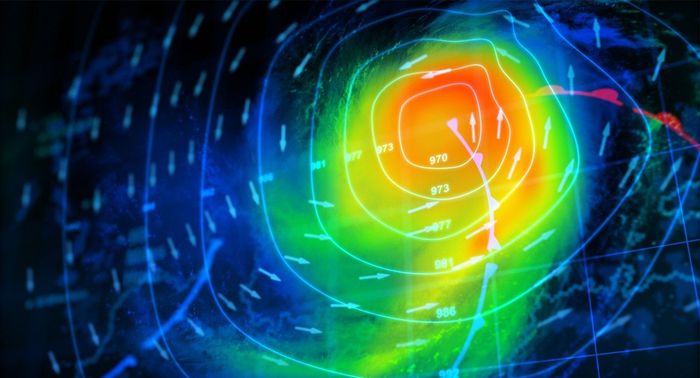
This model performs exceptionally well in almost all weather conditions, except for locally formed phenomena, i.e., at small scales. Prime examples are storms that initially form at a small scale and grow over time.
To better support forecasting 'hard cases', experts have applied machine learning algorithms, starting with the 'random forests' algorithm utilizing 'Decision Tree' structures to divide large datasets acquired from weather stations and automatically predict. Essentially, this tool still operates based on traditional weather prediction methods but provides more comprehensive information, including analyzing whether rainfall is likely to cause flooding.
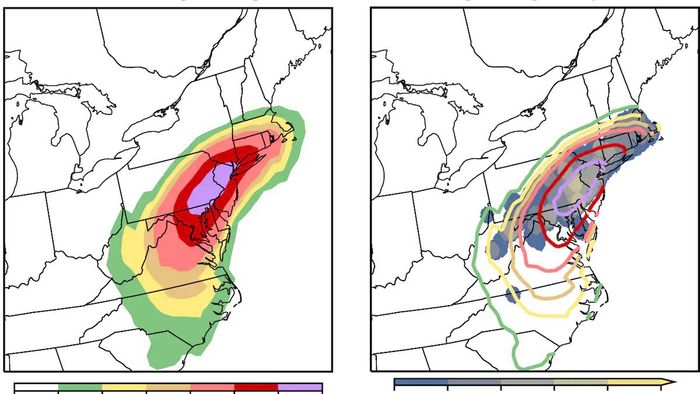
In addition to rain, this method is also highly effective in predicting tornadoes, hail, and hurricane winds. Furthermore, in some forecasting units, this tool has been elevated to a new 'height', specifically, the US National Weather Service relies on AI to provide conclusions on hazardous weather for daily warnings.
Moreover, machine learning processes assist humans in calculating weather data faster and smarter. For instance, through water vapor data, conclusions about whether there will be rain, snow, or hail can be quickly and extremely accurately drawn, saving time and ensuring precision.
Machine learning algorithms and AI hold great potential in meteorological forecasting. Hence, several studies have been conducted to ascertain whether they are the perfect replacement for traditional computation-based prediction methods or not. And the answer is no, at least not at present; intelligent algorithms can only predict general signals and cannot provide clear details about local weather conditions.
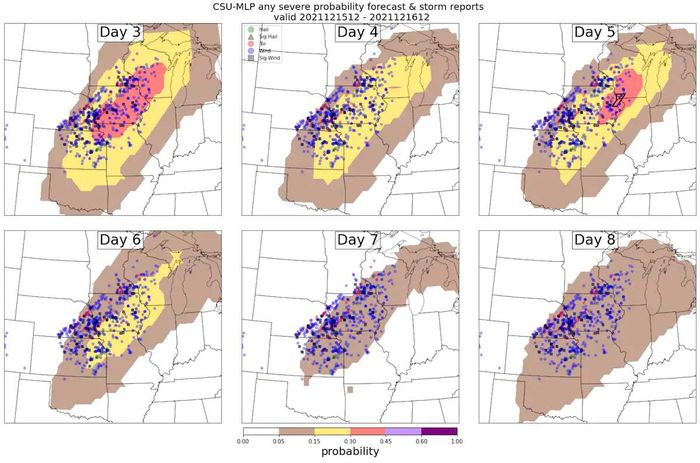
To provide a visualization, above illustrates the machine learning algorithm's forecasting process in December 2021 to analyze the likelihood of adverse weather phenomena. It covers 8 days before the occurrence (bottom right) to 3 days before the occurrence (top left), showing that the closer to the disaster day, the newer algorithms predict (red indicates tornadoes, blue denotes thunderstorms, and green signifies hail).
It takes a whopping 3 days to predict disasters, while 3 days pose challenges in thorough disaster preparedness, leaving only time for evacuations, with properties bearing the brunt. Like Typhoon Noru's recent abrupt appearance in the Philippines causing significant property losses, home destruction, and heavy agricultural damage.
The limitations of AI and the 'brainpower' of humansAs seen above, the current machine learning weather prediction speed only reaches a 'rough' level, not swift enough for coping and not superior enough to replace traditional computational methods. Fundamentally, current machine learning cannot yet capture all the physical laws affecting the atmosphere—a potent factor influencing weather phenomena.
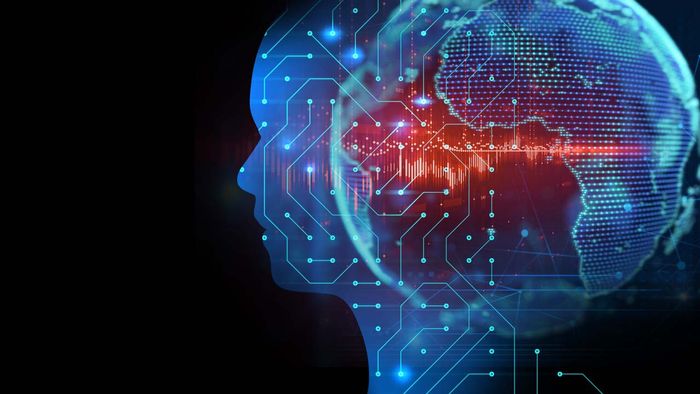
Hence, algorithms may 'misfire' compared to what will actually occur, for instance, based on gradually increasing temperature information (e.g., a 1-degree increase in a day), machine learning may predict a temperature beyond the usual tolerance level through gathered information, such as forecasting a scorching 100°C (based on temperature rise). Who would endure that?
Such mispredictions are more likely in unpredictable weather or unfamiliar phenomena, leading to encountering high false alarms generated by machine learning. Thus, human vigilance is necessary.
Furthermore, overly relying on AI and machine learning algorithms can raise concerns about resource wastage. Applying machine learning and training them without yielding significant efficiency while incurring costs for 'training' AI and algorithms for each specific region.
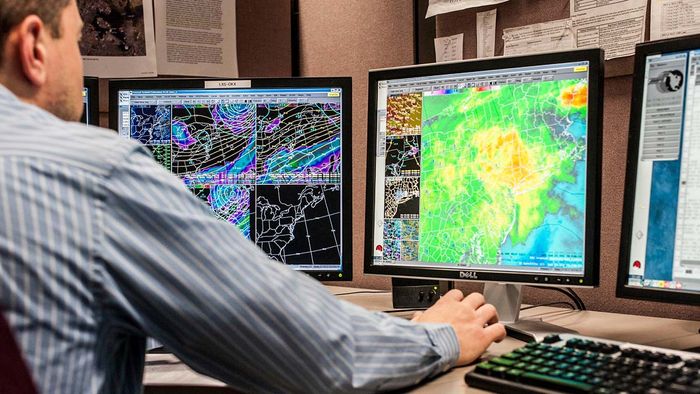
This leads to another consequence; weather forecasting units will face challenges in resource optimization, balancing between automated AI forecasts and weather forecasting knowledge from experts. If machine learning tools and AI are not applied to weather forecasting early, it's not conducive to advancing predictive technologies. However, insistence on relying solely on predictive tools will affect the current workflow of an organization.
Therefore, currently, AI still plays a role as a tool, to enable faster predictions by experts, rather than independently predicting due to the issues mentioned above. Thanks to AI, forecasters will have more time to communicate, exchange information with the public to provide early warnings.
Humans remain the key factorCurrently, sudden storms or unforeseen weather phenomena are always a challenge for AI because weather prediction is a combination of many aspects: observation, data computation, physics, and weather cycles. These are not just day-to-day data that AI can collect and predict, hence the key role of humans remains pivotal. Perhaps we need more time for AI weather forecasting to be perfected.
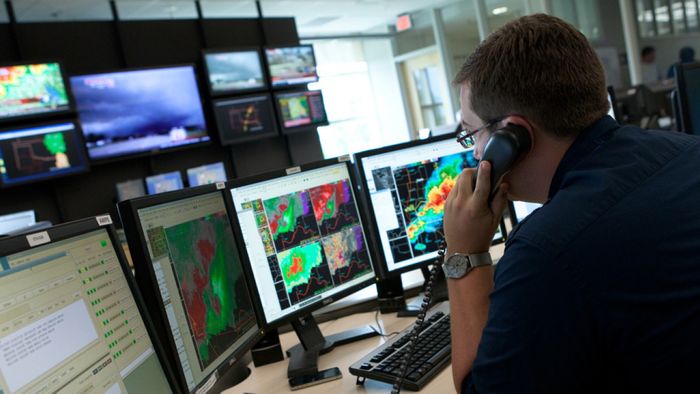
Perhaps the most beneficial contribution of AI to the weather forecasting industry is advertising. Channels like The Weather Channel, the primary source of default weather forecasts on most apps, utilize AI to deliver weather-appropriate advertisements to optimize profits. In this aspect, AI and machine learning excel, just look at Facebook.
- Explore more articles in the Discovery category